Introduction: In the era of COBOL, business rules and decisions were intricately woven into the fabric of the program itself. As COBOL programming methodologies advanced, subroutines and copybooks became popular tools for organizing and reusing code. However, even minor changes required the program to be recompiled, a time-consuming and resource-intensive process.
The landscape stayed relatively the same with the rise of client-server technologies, with C, C++, or Java becoming the preferred programming languages. While these languages offered improvements in terms of efficiency and versatility, the need for human intervention in the coding process remained constant.
A significant paradigm shift occurred with the inception of rules engines. These innovative tools enabled the externalization of rules and decision trees, allowing for dynamic invocation and greatly enhancing the flexibility of programming. Yet, despite this advancement, the responsibility of deliberating, deciding, and coding the necessary rules still fell on the shoulders of human domain experts and programmers.
The game’s rules are being rewritten with the advent of Generative AI (Gen AI). Gen AI has the potential to build and modify rules dynamically as new data becomes available, marking a departure from traditional methods. This shift heralds a new era in decision-making models, transitioning from a ‘human-centric’ approach to a ‘data-centric’ one.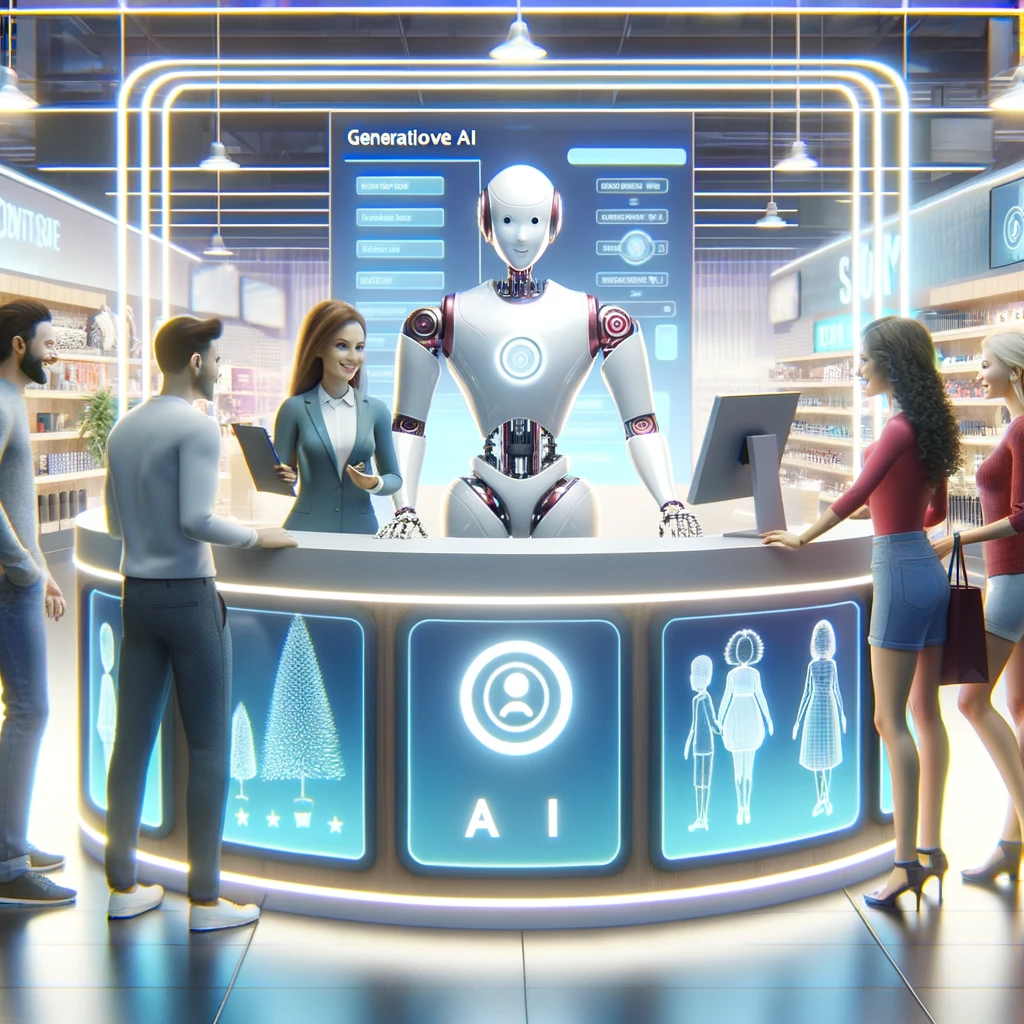
The Impact of Gen AI on Recommendation Engines:
Integrating Gen AI into recommendation engines promises to bring about several transformative changes. These include:
Revolutionizing Offers, Deals, and Promotions: Businesses can transition from human-centric decision models to data-centric ones by leveraging Gen AI. This shift allows for more personalized and dynamic offers, deals, and promotions tailored to user preferences and behaviors.
Reducing Effort in Analysis and Reviews: With Gen AI, the need for extensive analysis and reviews of decision models can be significantly reduced. The AI can automatically learn from the data, identify patterns, and make decisions, thereby streamlining the process.
Automating Rules Generation and Execution: Gen AI can automate the process of rules generation and execution. This automation increases efficiency and reduces the potential for human error.
Automating Coding: Gen AI can automate the coding process, further reducing the need for human intervention and accelerating the development cycle.
Benefits: Gen AI provides businesses with the ability to enhance their recommendation engines, resulting in a range of benefits:
Hyper-personalization: Businesses can deliver tailored recommendations and offers aligned with individual interests and requirements by analyzing customer data in detail.
Real-time Adaptability: Businesses can respond to changing customer behavior and market trends in real time, ensuring that their offers and promotions remain relevant and practical.
Elevated Customer Experience: By providing personalized interactions, businesses can delight customers, demonstrate understanding of their preferences, and establish brand loyalty while increasing conversion rates.
Challenges: Gen AI in recommendation engines presents unique challenges that must be addressed for effective implementation:
Data Integrity and Bias: Gen AI models must be trained on diverse, high-quality datasets to avoid biased or inaccurate recommendations. Continual monitoring and refinement are crucial.
Explainability: While Gen AI can automate decisions, understanding the reasoning behind recommendations is essential. Techniques to ensure explainability build trust and aid in troubleshooting.
System Integration: Incorporating Gen AI into existing processes often requires significant changes. A smooth transition requires a thorough impact analysis of systems, finances, and organizational structure.
Hallucinations: Gen AI models can sometimes produce incorrect or misleading outputs. Robust safeguards and quality control processes are needed to mitigate these “hallucinations.”
The example of Google Gemini’s inaccurate and offensive image generation underscores the importance of ensuring data quality and model explainability to maintain confidence and protect organizational reputation.
Conclusion: Implementing Gen AI in programming and decision-making processes is a significant advancement that can increase efficiency and improve personalization while decreasing human intervention. However, it is crucial to carefully consider the quality of data used, the ability to explain the decision-making process, and the overall impact on the organization to take advantage of its benefits and address its challenges fully.
Although Gen AI technologies are currently in their early stages, they are rapidly developing and hold immense potential for the future. These technologies can significantly reshape decision-making models and recommendation engines in the coming months. This will bring about a new era of automated, data-driven decision-making. As we continue to explore and harness the power of Gen AI, we can expect to see more efficient, accurate, and personalized recommendation engines than ever before.